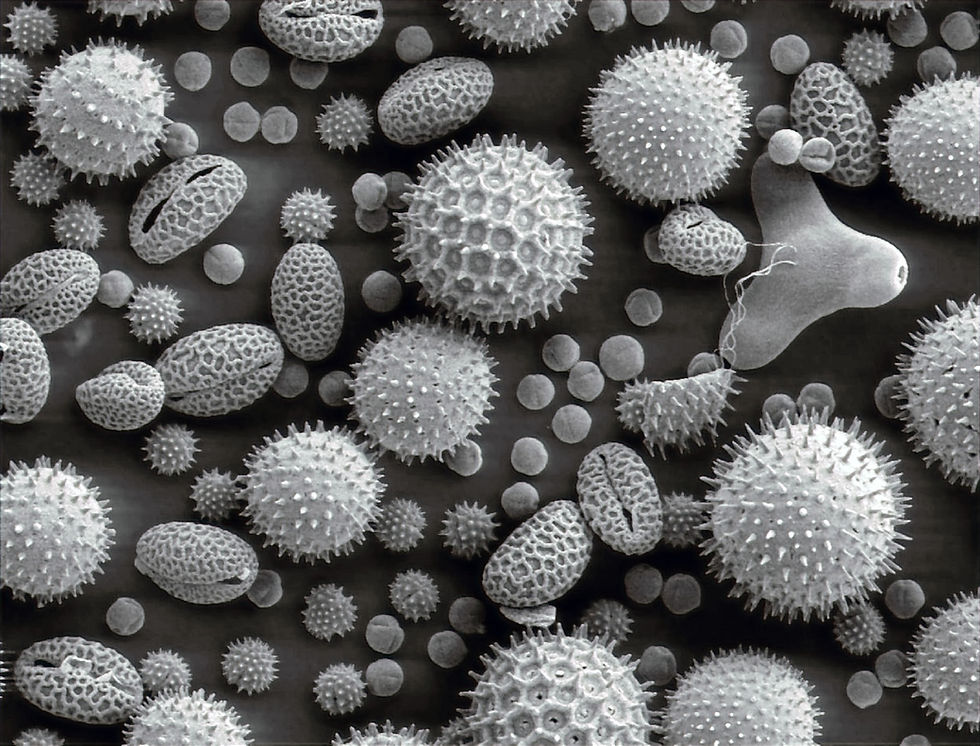
Consider a biological sample, for example pollen on an insect body. If you aim at sorting the pollen’s species according to their abundance, you will inevitably find that some pollen is super-dominant (i.e. very abundant) and other pollen is extremely rare, with a gradient in between. So far so good, but if you calculate any diversity statistics, the chosen metric will be influenced by the very abundant taxa which will shadow the other diversity. There are many ways to possible handle or correct for this, but I will not deal with it now. Another face of the problem comes from species that are very rare in the samples. Are these less abundant ones biologically reliable? Say, a bee collected two pollen grains from plant #1 and 100 from plant#2, could we confidently say the bee collects heterogeneous resources? Or would rather be better to ignore the 2 grains of species #1 (that resulted of stochastic event? Or from cross contamination between flowers?)? I would go for excluding the two grains… but the same issue arises from DNA data. In recent times, pollen have been identified with DNA metabarcoding, probably the best way to identify at species level given the difficulties at sorting species of pollen morphologically. The output of these type of data are matrices where sequenced pollen species are associated to a given number of DNA sequencing “reads” for each sample, that are quantitative numbers saying how much DNA you got from your DNA analysis protocol. Well, again, some species will be very rare, others will not be. How to behave? Some suggestions would be to remove everything below 1% of the reads abundance, while others would suggest to use the number of reads resulted from sequencing blanks (that are empty vials put in the machine to calculate possible machine-related contamination). While the second option seems very reasonable, it ignores the differences between species in amplification procedure: you could get few reads because a species has very thick pollen walls and hardly released any DNA; if so, should it be excluded because it resulted less abundant than a blank? Maybe not. On the other hand, using a fixed 1% threshold ignores the read counts distribution in the samples: say you have one species with 98% of the pollen and three species with about 0.66% of the reads, should we exclude the three? With such skewed distribution using fixed threshold is very risky. That is why I usually prefer using a ROC threshold calculation procedure. It is very simple but robust and everyone loves it, yet it is hardly ever used in ecological or DNA data.
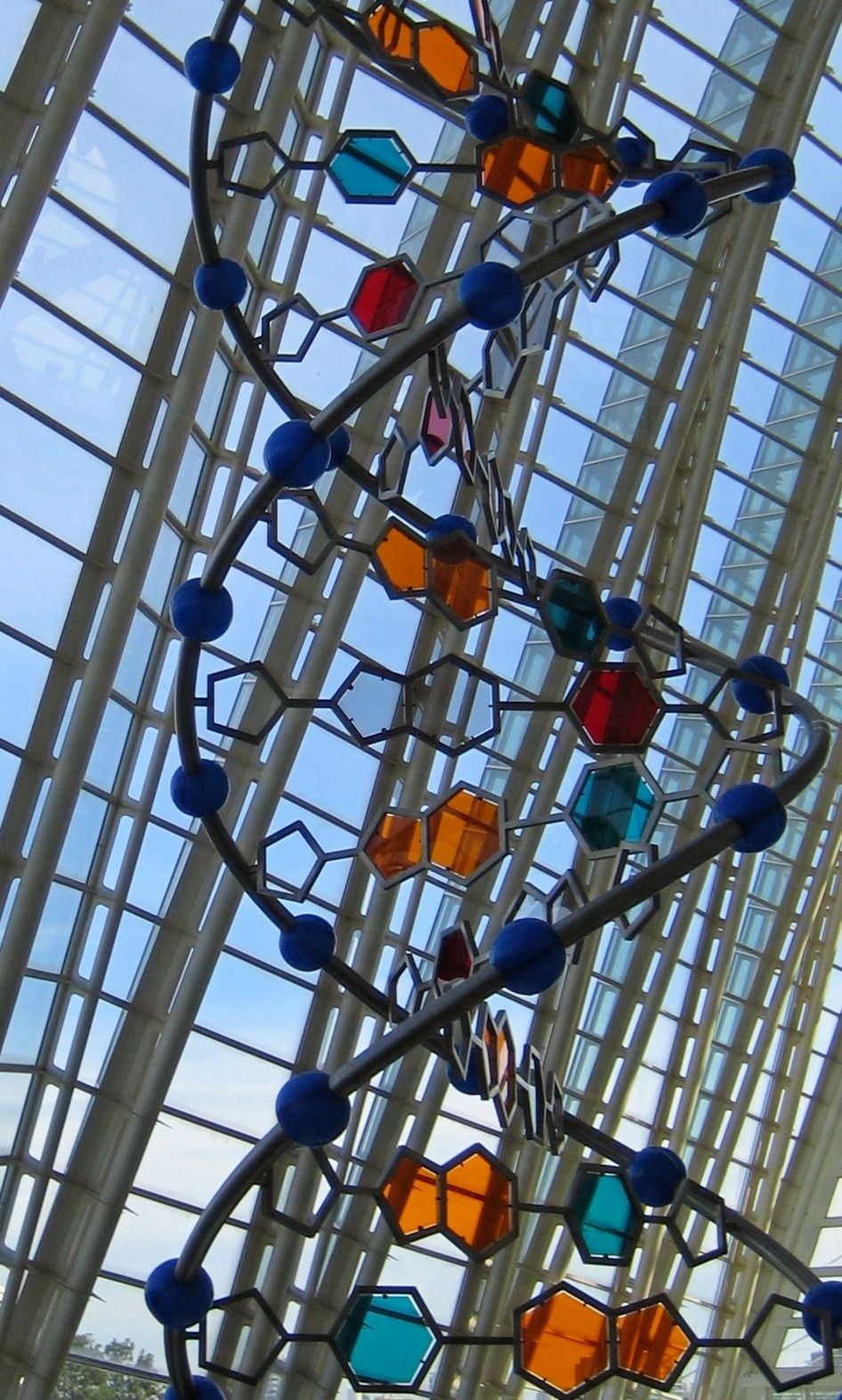
The procedure consists in statistically estimating the rate of false and true positives and negatives for each sample by associating the number of reads to these categories, and thus obtaining a sample-dependent threshold based on how the reads are actually distributed in your sample (b.t.w., you can also obtain a cross-samples threshold if you prefer having a constant one). I used this approach in both a pollen based study (see here!) and a microbe-based study (in revision), and the results always make more sense to me than using other cleaning approaches (the above mentioned ones) that basically clean too much, or not cleaning at all, where you risk to have too much diversity involved and you would dilute the important information in a mess of data. However, the possible solution is to try different approaches and decide according to the data you have, because sometimes even ROC cleans “too much”.
The figures are about pollen (the first) and a reconstructed DNA molecule (the second), are taken from Pxfuel and hold a CC licence.
Comentários